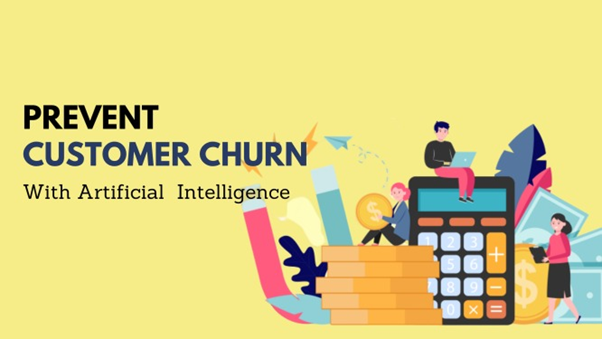
The banking industry has gone through a steady digital transformation over the years, but rising customer attrition rates are a concern now more than ever. The ever-increasing competition from existing players, neo banks like Monzo, N26, etc, and BigTech like Google, Amazon entering the sector, along with changing customer expectations have forced the Banks to rethink their strategies on customer retention and loyalty.
According to a recent survey, 67% of the unhappy customers state “poor service” as the major reason why they switch banks.
Data suggests that the cost of acquiring a new customer is 5X times more than retaining an existing one. The average revenue per user (ARPU) is much higher for existing customers who contribute more than 70% to overall revenues when compared to new customers.
“How can banks identify “at-risk customers” and “prevent” customer churn before it happens?”
First, let's look at the identification of “at-risk customers”. A good starting point is to analyze the churn rate/Dormancy data for the last 3-5 years.
Is the annual churn rate under 10%, 20%, or more?
What’s the churn trigger/pattern e.g. onboarding dropout?
Is a particular product/service skewing the attrition rate e.g. a co-branded credit card?
Is churn concentrated in a particular segment e.g. HNW?
Consumer behaviour is complex and there are multiple patterns involved in the accurate identification of micro-level parameters which lead to the identification of “at-risk customers”.
Is analyzing the customer churn data and having a reactive approach enough in 2020?
While there are plenty of churn analysis systems based on statistical models like RFM (recency, frequency, monetary), logistic regression, etc., where human intervention may or may not happen at the right time. Predicting and preventing customer churn should happen simultaneously for real proactive retention.
Predicting churn is not enough to prevent churn.
It’s time to move to Insight based action using predictive models that help you predict the churn risk/risk of dormancy at the individual customer level and take proactive action to prevent churn.
How can you leverage Comprehensive AI for automated churn prevention?
For effective churn prevention, you need to truly “understand” your customer, “identify” their financial journey, and “predict” best action in that micro-moment.
AI can be used to create an in-depth view of each customer based on all available customer data, including their account transactions, credit history, spending patterns. Using this information it becomes possible to provide customized offers and micro-targeted, truly personalized recommendations to each customer, through their preferred channels.
Comprehensive AI acts as a glue to help banks create a holistic view of each customer, accurately pinpoint the underlying causes of customer churn across various touchpoints, products, segments, behaviours, demographics, geographies et al.
Let’s see the three pillars of AI for churn prevention for banks:
Dynamic Customer persona : Machine learning models and big data analytics can help create dynamic customer personas and perform real-time peer persona mapping to identify customers showing behaviour similar to those who churned in the past. Leverage AI to predict churn-like events by learning from patterns in customer switching behaviour even before an at-risk customer starts showing signs of attrition and uses reinforcement learning to enhance the ML model with the result of the recommendation set.
Sentiment analysis : Sentiments are mainly inferred from the vast unstructured data points collected from customer interactions at various touchpoints e.g. contact center logs. Identifying the “unhappy” signs and proactively moving them in priority and offering them hyper-personalized recommendations can help turn them around.
Transaction pattern analysis : Banks can leverage AI to analyze vast amounts of transactional data of their customers including deposits, withdrawals, credit card activities, and payments and understand patterns. These transactional patterns are used to reveal any unusual customer behaviours, early-warning signs, and automate NBA (Next Best Action) and personalized recommendations.
Success story
- Proactive service - Delight “at-risk” customers with automatically upgraded service across channels e.g. Call/Chat routing to senior team members or retention SWAT team.
- Personalized offers - Leverage hyper-personalized offers for own and third-party products to increase the relationship value e.g. offers on concert tickets for musically inclined.
- Human-in-the-loop -Timely action recommendation to RM/Service desk to engage customers to prevent attrition.
With a strong domain-specific cognitive model, BankBuddy equips you to leverage AI and behavioural analytics to accurately predict and prevent churn by offering hyper-personalized banking experiences to your at-risk customers.
Read more about how we create dynamic customer personas for hyper-personalised banking experience https://bankbuddy.ai/hyper-personalize-banking-with-recommendation-engine